You are excited about your new design. It certainly looks more beautiful than the production, but when it comes time to A/B test, it doesn’t fare well. Key metrics drop and your craft cannot be shipped.
If you’re a product designer, does this resonate with you? Has something like this happened to you before?
Evaluating designs and making shipping decisions based on data is a common practice in tech companies, and it has a big impact on design outcomes. Booking.com and Amazon are well known for A/B testing their designs extensively and picking the best performing one in metrics to maximize their profit.
Data-driven design can be tricky. To succeed, we need to be strategic.
Design and Metrics
User engagement is key to the survival of a business, and tech companies develop sophisticated systems to measure it.
When design is data-driven, its success hinges on metrics such as click-through rate, impressions, and dwell time. Because layouts, colors, and positions of UI elements can influence how users engage with a product, design decisions are often made to optimize the metrics. Product managers like to quote the gain or improvement in these metrics to say they can roll out this feature with this design because it will have a positive impact on the business.
To minimize risks and maximize the metric gain, product teams usually A/B test multiple designs. The metrics of these designs will then be compared, and the best performing design will be shipped.
Two Scenarios
There are two scenarios in data-drive design. In the first one, we’re designing for a new feature. Shipping a design like this tends to be relatively easy, as new features usually create new engagement opportunities and therefore increase metrics.
What’s harder and more tricky to handle is the second scenario, where we’re creating a new design for an existing feature. If the current design already performs quite well, it can be quite challenging for a new design to work even better.
Observe and Analyze
When the second scenario occurs, the first thing we should do is to observe and analyze the existing design and see how it achieves the current level of engagement: What draws user attention in the design? What affordances are created here? What cognitive biases are leveraged?… Then we form hypotheses and hopefully leverage them in our new design.
Let’s say the existing design is a carousel of densely packed image cards. By analyzing it, we hypothesize that its high density and the affordance that invites users to click that’s created by lining up items in a single row, contribute to its current performance in metrics.

Doing this kind of dissection is crucial. If we skip it, our new design could easily lose the metrics game.
Because the existing design already has pretty good metrics, one possible way to beat it is to build upon it. Compared with coming up with a brand new design, it’s probably a safer path that can lead to a shippable outcome.
Progressive Changes
As you can see, data-driven design is all about the relationship between design and metrics. To get more clarity, we can also apply the principle of progressive changes. The idea is to insert one or two intermediate versions with less dramatic changes between the current design and the new one we create during an A/B test. How granular those changes are will be determined by the complexity of the new design and the goal of the test. By introducing changes in small batches, we can gain pretty good insights into how design actually impacts metrics.
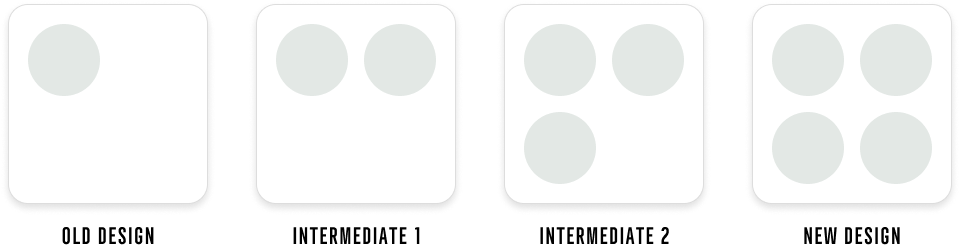
Limitations of Data-Driven Design
Depending on the culture of the team or company, sometimes the success of our design can solely depend on the outcome of quantitative metrics. The goal a design needs to achieve therefore is shifted. Even if our design is beautiful and well received during user tests, if its metrics are not good, then there are likely obstacles to shipping it. Design, in this case, is reduced to a number game.
This is where this approach could be a bit rigid or even misleading sometimes, especially when higher metrics are blindly pursued without thoughtful consideration of the entire user experience.
Let’s say the same content is displayed twice on a page, one at the top, the other at the bottom, purely for the purpose of increasing its exposure. We may record more clicks during an A/B test, however, is this really good UX? Won’t users be able to realize that the repeating content is a trick?
UX is not numbers. It evokes emotions, gut reactions and even thoughts from the user. No matter how Booking.com A/B tests their designs, if they can’t go beyond the numbers, if they don’t think about their UX differently, they won’t be able to offer the kind of pleasant experience delivered by the clean and beautiful design of AirBnB. Or think about Google’s homepage. If they fill the generous amount of whitespace surrounding the search box with content, click-through rate or dwell time on that page probably will rise in an A/B test. However, Google doesn’t succumb to the seduction of short-term gain in metrics. They stick to their minimal design. The result is a clean, efficient, non-distracting user experience for search, which is loved by users.
Final Thoughts
To reach a shippable result faster in data-driven design, observe and analyze the relationship between design and metrics carefully, and introduce changes progressively in an A/B test. Keep in mind that data-driven design has its limitations. A good product designer thinks about UX holistically, and won’t let metrics alone cloud his or her judgement.